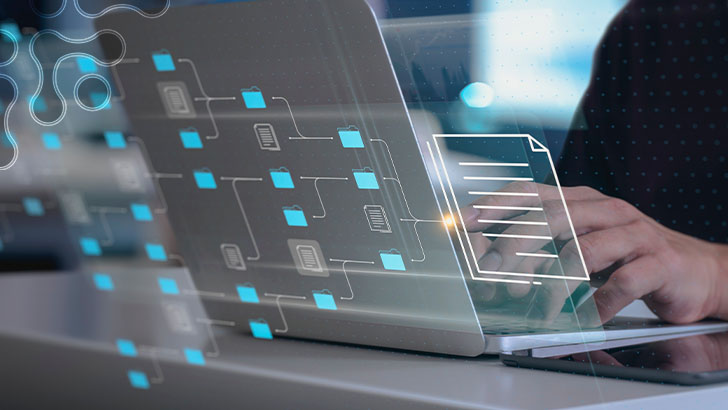
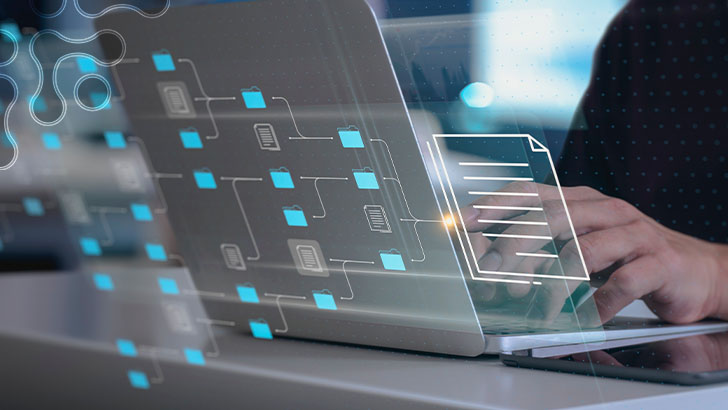
The aim of this topic is to harness advanced artificial intelligence/machine-learning (AI/ML) technologies to enhance The Integrated Personnel and Pay System- Army (IPPS-A) by achieving the following objectives:
AI/ML is best suited to tackle these issues over traditional software approaches because the tool can be trained to identify common and new issues with data. Finding incongruencies in our data requires manual intervention and that requires time and money.
We want to reduce or eliminate the time it takes to triage/adjudicate data quality issues and AI/ML can do this instantaneously and accurately. An AI/ML tool can scan an entire data set for anomaly identification while manual means relies on specific data attributes.
The Army has massive data quality issues going back decades. IPPS-A inherited quite a bit of incomplete or incorrect data, which does not function well in a modern system with data quality checks. There were minimal data completeness or correctness checks in legacy systems, and Soldiers could have a record in more than one of the four legacy databases in some degree of currency.
IPPS-A converted 1.1 million records from those four databases and made some decisions on what was coming from where, or with our partners who are relying on complete, correct data from us. A human captures datapoints from paper documents to establish personal identity data and service data in accessions systems, which pass data to IPPS-A. IPPS-A is building a payroll system bringing data from legacy systems to the functioning HR system.
This solution would improve the Service Members quality of life by delivering accurate pay, reduced interactions with HR and Pay Administrators on data issues, increase the reliability on the program, and concentrate on duty tasks more efficiently. Normal data quality implementations check and validate specified data fields through a manual process that takes time, whereas an AI driven anomaly detection could look at the entire data set from an inbound interface system and within the IPPS-A system.
We want to introduce AI/ML driven anomaly detection to IPPS-A. This capability would help users at multiple levels even though the improvement is not outward facing and noticeable to end-users.
This topic is only accepting Direct to Phase II proposals for a cost up to $2,000,000 for a 24-month period of performance. Proposers interested in submitting a DP2 proposal must provide documentation to substantiate that the scientific and technical merit and feasibility equivalent to a Phase I project has been met. Documentation can include data, reports, specific measurements, success criteria of a prototype, etc.
Improve the Service Members’ quality of life by delivering accurate pay, reduced interactions with HR and Pay Administrators on data issues, increase the reliability on the program and concentrate on duty tasks more efficiently.
Speaking with multiple vendors about data quality improvement utilizing AI/ML is completely possible and being done today. Companies are eager to solve this problem and want to be a part of improving the Army for everyone.
Justify that the enabling technologies are mature enough to position a diverse set of companies to deliver a prototype via a Direct to Phase 2, instead of initiating this topic with a Phase 1 feasibility study.
Data quality improvement solutions can span across many organizations. For example, if companies merge or acquired, the parent company can utilize data quality improvement solutions to optimize their HR and payroll data.
For more information, and to submit your full proposal package, visit the DSIP Portal.
SBIR|STTR Help Desk: usarmy.sbirsttr@army.mil
References:
The aim of this topic is to harness advanced artificial intelligence/machine-learning (AI/ML) technologies to enhance The Integrated Personnel and Pay System- Army (IPPS-A) by achieving the following objectives:
AI/ML is best suited to tackle these issues over traditional software approaches because the tool can be trained to identify common and new issues with data. Finding incongruencies in our data requires manual intervention and that requires time and money.
We want to reduce or eliminate the time it takes to triage/adjudicate data quality issues and AI/ML can do this instantaneously and accurately. An AI/ML tool can scan an entire data set for anomaly identification while manual means relies on specific data attributes.
The Army has massive data quality issues going back decades. IPPS-A inherited quite a bit of incomplete or incorrect data, which does not function well in a modern system with data quality checks. There were minimal data completeness or correctness checks in legacy systems, and Soldiers could have a record in more than one of the four legacy databases in some degree of currency.
IPPS-A converted 1.1 million records from those four databases and made some decisions on what was coming from where, or with our partners who are relying on complete, correct data from us. A human captures datapoints from paper documents to establish personal identity data and service data in accessions systems, which pass data to IPPS-A. IPPS-A is building a payroll system bringing data from legacy systems to the functioning HR system.
This solution would improve the Service Members quality of life by delivering accurate pay, reduced interactions with HR and Pay Administrators on data issues, increase the reliability on the program, and concentrate on duty tasks more efficiently. Normal data quality implementations check and validate specified data fields through a manual process that takes time, whereas an AI driven anomaly detection could look at the entire data set from an inbound interface system and within the IPPS-A system.
We want to introduce AI/ML driven anomaly detection to IPPS-A. This capability would help users at multiple levels even though the improvement is not outward facing and noticeable to end-users.
This topic is only accepting Direct to Phase II proposals for a cost up to $2,000,000 for a 24-month period of performance. Proposers interested in submitting a DP2 proposal must provide documentation to substantiate that the scientific and technical merit and feasibility equivalent to a Phase I project has been met. Documentation can include data, reports, specific measurements, success criteria of a prototype, etc.
Improve the Service Members’ quality of life by delivering accurate pay, reduced interactions with HR and Pay Administrators on data issues, increase the reliability on the program and concentrate on duty tasks more efficiently.
Speaking with multiple vendors about data quality improvement utilizing AI/ML is completely possible and being done today. Companies are eager to solve this problem and want to be a part of improving the Army for everyone.
Justify that the enabling technologies are mature enough to position a diverse set of companies to deliver a prototype via a Direct to Phase 2, instead of initiating this topic with a Phase 1 feasibility study.
Data quality improvement solutions can span across many organizations. For example, if companies merge or acquired, the parent company can utilize data quality improvement solutions to optimize their HR and payroll data.
For more information, and to submit your full proposal package, visit the DSIP Portal.
SBIR|STTR Help Desk: usarmy.sbirsttr@army.mil
References: